9.3 Speech Production Models
The Dell Model
Speech error analysis has been used as the basis for the model developed by Dell (1986, 1988). Dell’s spreading activation model (as seen in Figure 9.3) has features that are informed by the nature of speech errors that respect syllable position constraints. This is based on the observation that when segmental speech errors occur, they usually involve exchanges between onsets, peaks or codas but rarely between different syllable positions. Dell (1986) states that word-forms are represented in a lexical network composed on nodes that represent morphemes, segments and features. These nodes are connected by weighted bidirectional vertices.
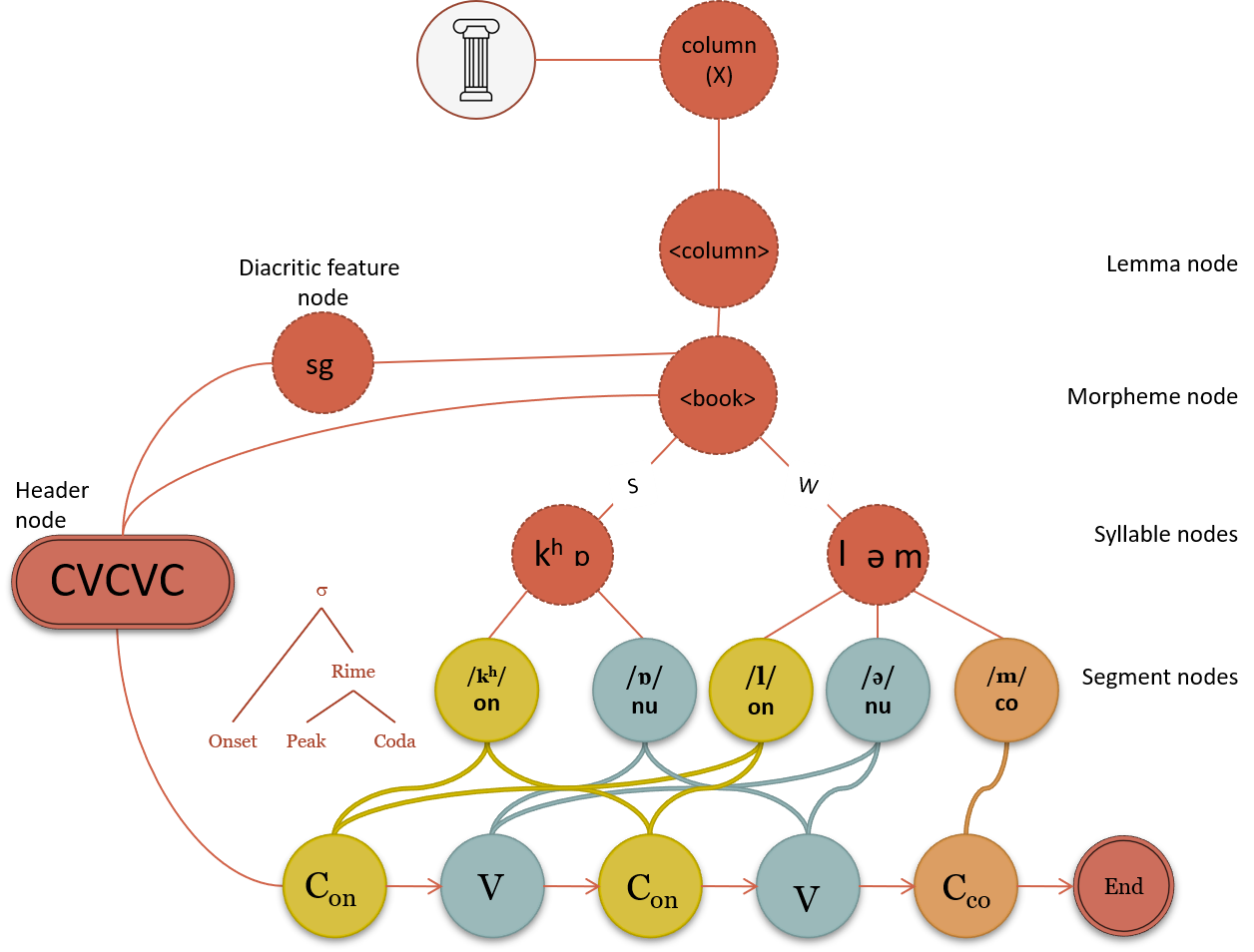
As seen in Figure 9.3, when the morpheme node is activated, it spreads through the lexical network with each node transmitting a proportion of its activation to its direct neighbour(s). The morpheme is mapped onto its associated segments with the highest level of activation. The selected segments are encoded for particular syllable positions which can then be slotted into a syllable frame. This means that the /p/ phoneme that is encoded for syllable onset is stored separately from the /p/ phonemes encoded for syllable coda position. This also accounts for the phonetic level in that instead of having two separate levels for segments (phonological and phonetic levels), there is only one segmental level. In this level, the onset /p/ is stored with its characteristic aspiration as [ph] and the coda /p/ is stored in its unaspirated form [p]. Although this means that segments need to be stored twice for onset and coda positions, it simplified the syllabification process as the segments automatically slot into their respective position. Dell’s model ensures the preservation of syllable constraints in that onset phonemes can only fit into onset syllable slots in the syllable template (the same being true for peaks and codas). The model also has an implicit competition between phonemes that belong to the same syllable position and this explains tongue-twisters such as the following:
- “She sells sea shells by the seashore” ʃiː sɛlz siːʃɛlz baɪ ðiː siːʃɔː
- “Betty Botter bought a bit of butter” bɛtiː bɒtə bɔːt ə bɪt ɒv bʌtə
In these examples, speakers are assumed to make errors because of competition between segments that share the same syllable position. As seen in Figure 9.3, Dell (1988) proposes a word-shape header node that contains the CV specifications for the word-form. This node activates the segment nodes one after the other. This is supported by the serial effects seen in implicit priming studies (Meyer, 1990; 1991) as well as some findings on the influence of phonological similarity on semantic substitution errors (Dell & Reich, 1981). For example, the model assumes that semantic errors (errors based on shared meaning) arise in lemma nodes. The word cat shares more segments with a target such as mat ((/æ/nu and /t/cd) than with sap (only /æ/nu). Therefore, the lemma node of mat will have a higher activation level than the one for sap creating the opportunity for a substitution error. In addition, the feedback from morpheme nodes leads to a bias towards producing words rather then nonword error. The model also takes into account the effect of speech rate on error probability (Dell, 1986) and the frequency distribution of anticipation-, perseveration- and transposition- errors (Nooteboom, 1969). The model accounts for differences between various error types by having an in-built bias for anticipation. Activation spreads through time. Therefore, upcoming words receive activation (at a lower level than the current target). Speech rate also has an influence on errors because higher speech rates may lead to nodes not having enough time to reach a specified level of activation (leading to more errors).
While the Dell model has a lot of support for it’s architecture, there have been criticisms. The main evidence used for the model, speech errors, have themselves been questioned as a useful piece of evidence for informing speech production models (Cutler, 1981). For instance, the listener might misinterpret the units involved in the error and may have a bias towards locating errors at the beginning of words (accounting for the large number of word-onset errors). Evidence for the CV header node is limited as segment insertions usually create clusters when the target word also had a cluster and CV similarities are not found for peaks.
The model also has an issue with storage and retrieval as segments need to be stored for each syllable position. For example, the /l/ in English needs to be stored as [l] for syllable onset, [ɫ] for coda and [ḷ] when it appears as a syllabic consonant in the peak (as in bottle). However, while this may seem redundant and inefficient, recent calculations of storage costs based on information theory by Ramoo and Olson (2021) suggest that the Dell model may actually be more storage efficient than previously thought. They suggest that one of the main inefficiencies of the model are during syllabification across word and morpheme boundaries. During the production of connected speech or polymorphic words, segments from one morpheme or word will move to another (Chomsky & Halle, 1968; Selkirk, 1984; Levelt, 1989). For example, when we say “walk away” /wɔk.ə.weɪ/, we produce [wɔ.kə.weɪ] where the /k/ moves from coda to onset in the next syllable. As the Dell model codes segments for syllable position, it may not be possible for such segments to move from coda to onset position during resyllabification. These and other limitations have led researchers such as Levelt (1989) and his colleagues (Meyer, 1992; Roelofs, 2000) to propose a new model based on reaction time experiments.
The Levelt, Roelofs, and Meyer (LRM) Model
The Levelt, Roelofs, and Meyer or LRM model is one of the most popular models for speech production in psycholinguistics. It is also one of the most comprehensive in that it takes into account all stages from conceptualization to articulation (Levelt et al., 1999). The model is based on reaction time data from naming experiments and is a top-down model where information flows from more abstract levels to more concrete stages. The Word-form Encoding by Activation and VERification (WEAVER) is the computational implementation of the LRM model developed by Roelof (1992, 1996, 1997a, 1997b, 1998, 1999). It is a spreading activation model inspired by Dell’s (1986) ideas about word-form encoding. It accounts for the syllable frequency effect and ambiguous syllable priming data (although the computational implementation has been more successful in illustrating syllable frequency effects rather than priming effects).
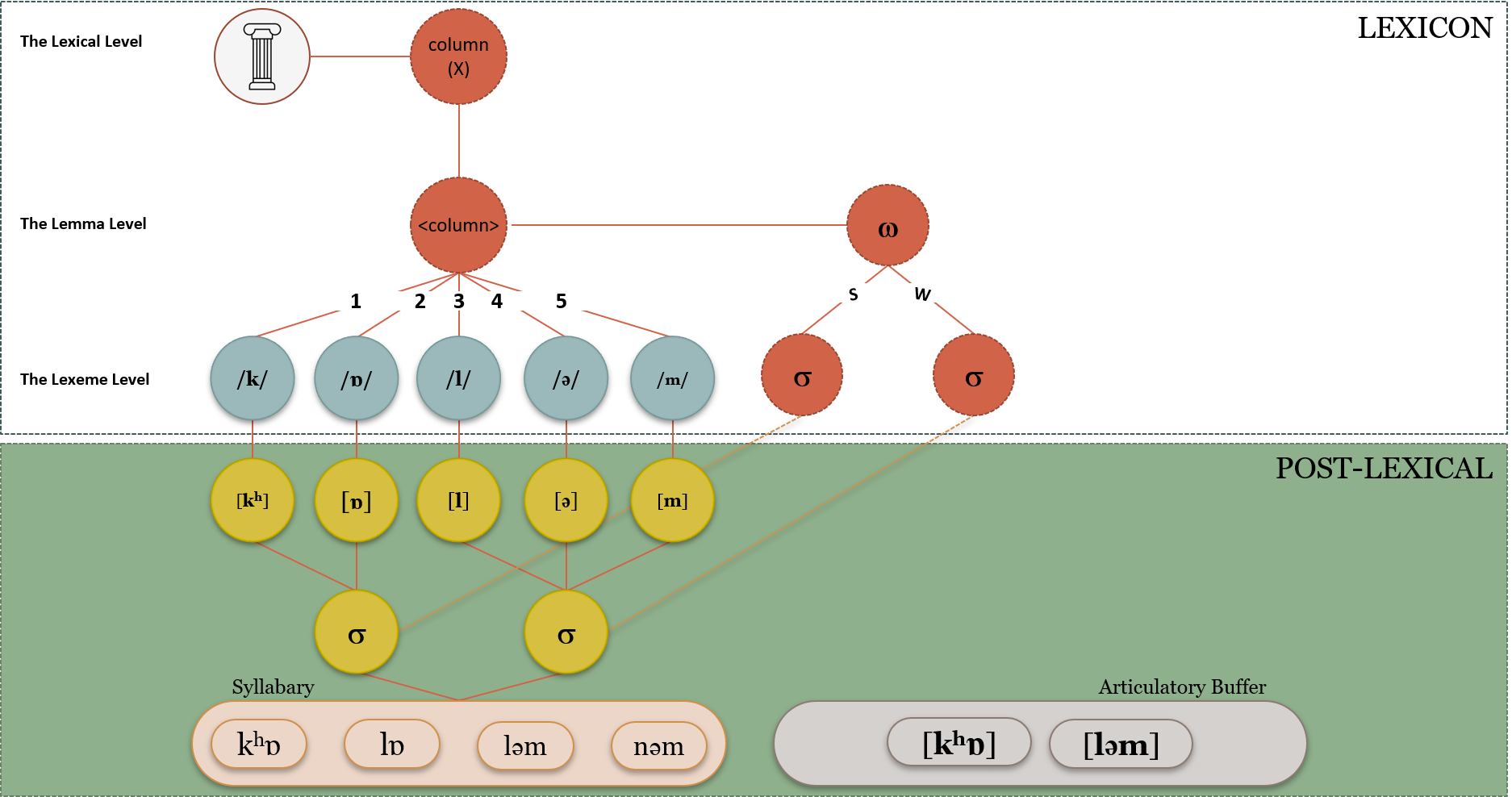
As we can see in Figure 9.4, the lemma node is connected to segment nodes. These vertices are specified for serial position and the segments are not coded for syllable position. Indeed, the only syllabic information that is stored in this model are syllable templates that indicate the stress patterns of each word (which syllable in the word is stressed and which is not). These syllabic templates are used during speech production to syllabify the segments using the principle of onset-maximization (all segments that can legally go into a syllable onset in a language are put into the onset and the leftover segments go into the coda). This kind of syllabification during production accounts for resyllabification (which is a problem for the Dell model). The model also has a mental syllabary which is hypothesized to contain the articulatory programs that are used to plan articulation.
The model is interesting in that syllabification is only relevant at the time of production. Phonemes are defined within the lexicon with regard to their serial position in the word or lemma. This allows for resyllabification across morpheme and word boundaries without any difficulties. Roelofs and Meyer (1998) investigated whether syllable structures are stored in the mental frame. They employed an implicit priming paradigm where participants produced one word out of a set of words in rapid succession. The words were either homogenous (all words had the same word onsets) or heterogeneous. They found that priming depended on the targets having the same number of syllable and stress patterns but not the same syllable structure. This led them to conclude that syllable structure was not a stored component of speech production but computed during speech (Choline et al., 2004). Costa and Sebastian-Galles (1998) employed a picture-word interference paradigm to investigate this further. They asked participants to name a picture while a word was presented after 150 ms. They found that participants were faster to name a picture when they shared the same syllable structure with the word. These results challenge the view that syllable structure is absent as an abstract encoding within the lexicon. A new model has challenged the LRM model’s assumptions on this with a Lexicon with Syllable Structure (LEWISS) model.
The Lexicon with Syllable Structure (LEWISS) Model
Proposed by Romani et al. (2011), the Lexicon with Syllable Structure (LEWISS) model explores the possibility of stored syllable structure in phonological encoding. As seen in Figure 9.5 the organisation of segments in this model is based on a syllable structure framework (similar to proposals by Selkirk, 1982; Cairns & Feinstein, 1982). However, unlike the Dell model, the segments are not coded for syllable position. The syllable structural hierarchy is composed of syllable constituent nodes (onset, peak and coda) with the vertices having different weights based on their relative positions. This means that the peak (the most important part of a syllable) has a very strongly weighted vertex compared to onsets and codas. Within onsets and codas, the core positions are more strongly weighted compared to satellite position. This is based on the fact that there are positional variations in speech errors. For example, onsets and codas are more vulnerable to errors compared to vowels or peaks. Within onsets and codas, the satellite positions are more vulnerable compared to core positions. For example, in a word like print, the /r/ and /n/ in onset and coda satellite positions are more likely to be the subjects of errors than the /p/ and /t/ which are core positions. The main evidence for the LEWISS model comes from the speech errors of aphasic patients (Romani et al., 2011). It was observed that not only did they produce errors that weighted syllable positions differently, they also preserved the syllable structure of their targets even when making speech errors.
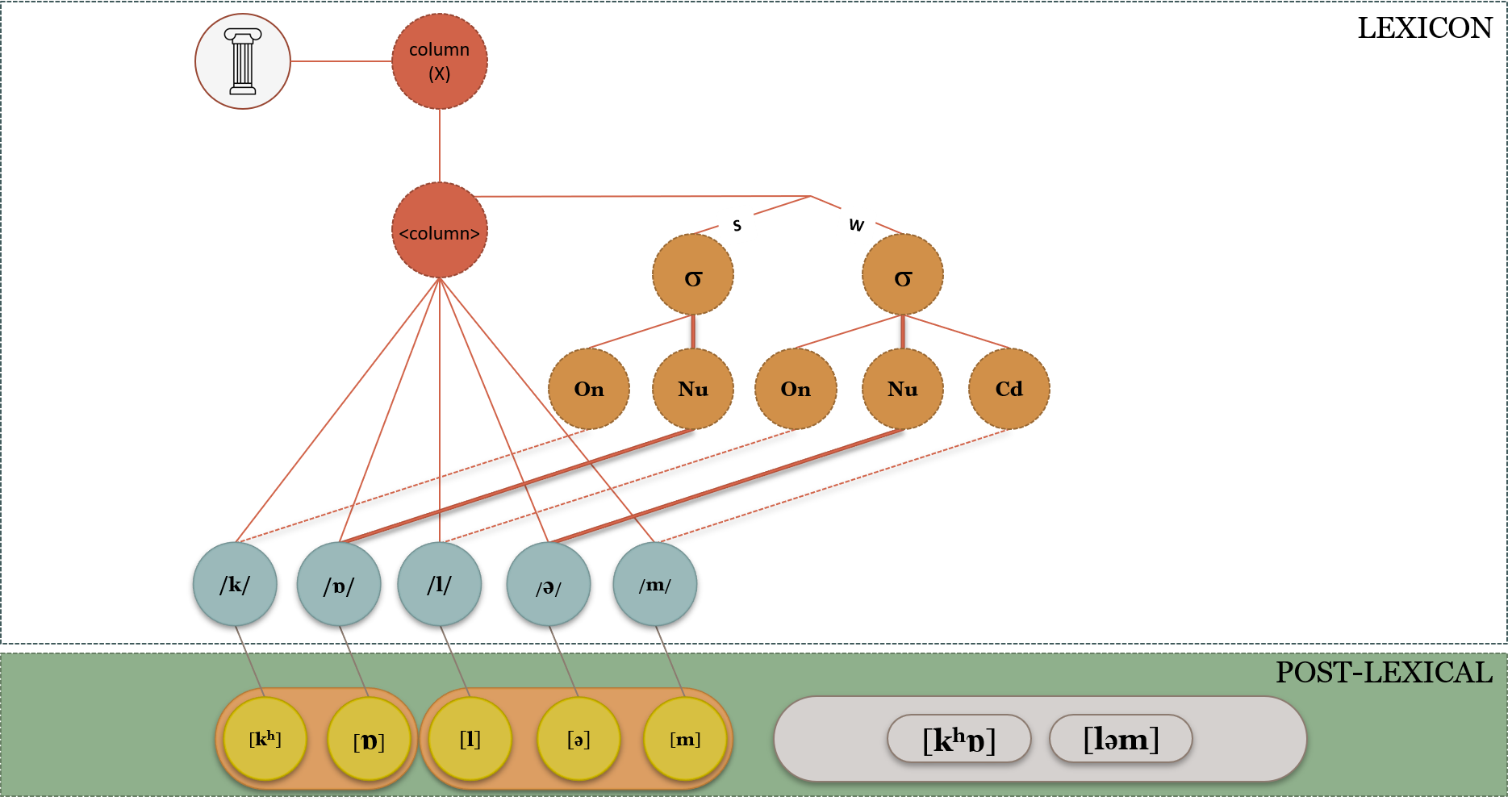
In terms of syllabification, the LEWISS model syllabifies at morpheme and word edges instead of having to syllabify the entire utterance each time it is produced. The evidence from speech errors supports the idea of having syllable position constraints. While Romani et al. (2011) have presented data from Italian, speech error analysis in Spanish also supports this view (Garcia-Albea et al., 1989). The evidence from Spanish is also interesting in that the errors are mostly word-medial rather than word-initial as is the case for English (Shattuck-Hufnagel, 1987, 1992). Stemberger (1990) hypothesised that structural frames for CV structure encoding may be compatible with phonological systems proposed by Clements and Keyser (1983) as well as Goldsmith (1990). This was supported by speech errors from German and Swedish (Stemberger, 1984). However, such patterns were not observed in English. Costa and Sebastian-Gallés (1998) found primed picture-naming was facilitated by primes that shared CV structure with the targets. Sevald, Dell and Cole (1995) found similar effects in repeated pronunciation tasks in English. Romani et al. (2011) brought these ideas to the fore with their analysis of speech errors made by Italian aphasic and apraxic patients. The patients did repetition, reading, and picture-naming tasks. Both groups of patients produced errors that targeted vulnerable syllable positions such as onset- and coda- satellites consistent with previous findings (Den Ouden, 2002). They also found that a large proportion of the errors preserved syllable structure even in the errors. This is noted by previous findings as well (Wilshire, 2002). Previous findings by Romani and Calabrese (1996) found that Italian patients replaced geminates with heterosyllabic clusters rather than homosyllabic clusters. For example, /ʤi.raf.fa/ became /ʤi.rar.fa/ rather than /ʤi.ra.fra/ preserving the original syllable structure of the target. While the Dell model’s segments coded for syllable position can also explain such errors, it cannot account for errors that moved from one syllable position to another. More recent computational calculations by Ramoo and Olson (2021) found that the resyllabification rates in English and Hindi as well as storage costs predicted by information theory do not discount LEWISS based on storage and computational costs.
Language Production Models
Dell Model
- Concept Node
- This is the non-verbal concept of the object that is elicited when we see a picture, read the word or hear it.
- Lemma Node
- An abstract conceptual form of a word that has been mentally selected for utterance.
- Morpheme Node
- The meaningful unit (or units) of the lemma attached to specific segments.
- Syllable Nodes
- Syllable nodes are created using the syllable template.
- Segment Nodes
- Segment nodes are specified for syllable position. So, [p onset] will be a separate segment from [p coda].
- Diacritic Feature node
- This node indicates that the word is singular.
- Header node
- This node specifies the CV structure and order of the word.
- Syllable template
- A syllable template is used in the syllabification process to indicate which segments can go where.
- Segment Category Nodes
- The segment category nodes are specified for syllable position. So, they only activate segments that are for onset, peak or coda syllable positions. Activation will be higher for the appropriate segment.
LRM Model
- Conceptual Node
- This is the non-verbal concept of the object that is elicited when we see a picture, read the word or hear it.
- Lemma Node
- An abstract conceptual form of a word that has been mentally selected for utterance.
- Morpheme Node
- The meaningful unit (or units) of the lemma attached to specific segments.
- Segment Nodes
- Segment nodes are connected to the morpheme node specified for serial position.
- Syllable Template
- The morpheme is connected to a syllable template that indicates how many syllable are contained within the phonological word. It also indicates which syllables are stressed and unstressed.
- Post-lexical Syllabification
- Post-lexical syllabification uses the syllable template to syllabify the phonemes. This is also the place where phonological rules can be implimented. For example, in English, unvoiced stops will be aspirated in output.
- Mental Syllabary
- Syllabified representations are used to access a Mental Syllabary of articulatory motor programs.
- Output
- The final output.
LEWISS Model
- Conceptual Node
- This is the non-verbal concept of the object that is elicited when we see a picture, read the word or hear it.
- Lemma Node
- An abstract conceptual form of a word that has been mentally selected for utterance.
- Morpheme Node
- The meaningful unit (or units) of the lemma attached to specific segments.
- Syllable Structure Nodes
- The syllable structure nodes indicate the structure of the word’s syllable structure. They also specify syllable stress or tone. In addition, the connections are weighted. So, core positions and peak positions are strongly weighted compared to satellite positions.
- Segment Nodes
- Segment nodes are connected to the morpheme node. They are also connected to a syllable structure that keeps them in place.
- Post-lexical Syllabification
- Post-lexical syllabification syllabify the phonemes at morpheme and word boundaries. This is also the place where phonological rules can be implimented. For example, in English, unvoiced stops will be aspirated in output.
- Output
- The final output.
Navigate to the above link to view the interactive version of these models.
Media Attributions
- Figure 9.3 The Dell Model by Dinesh Ramoo, the author, is licensed under a CC BY 4.0 licence.
- Figure 9.4 The LRM Model by Dinesh Ramoo, the author, is licensed under a CC BY 4.0 licence.
- Figure 9.5 The LEWIS Model by Dinesh Ramoo, the author, is licensed under a CC BY 4.0 licence.
The process of putting individual segments into syllables based on language-specific rules.
The process by which segments that belong to one syllable move to another syllable during morphological changes and connected speech.
The structure of the syllable in terms of onset, peak (or nucleus) and coda.